Jianfeng Zhou
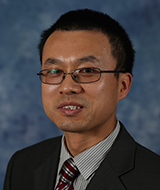
Assistant Professor
Plant Science and Technology
E-mail: zhoujianf at missouri dot edu
Office address: 211 Agricultural Engineering Building
Office phone: 573-882-2495
Jianfeng Zhou, PhD, an Assistant Professor of Agricultural Engineering at the Division of Plant Science and Technology of the University of Missouri (MU). He has primary teaching responsibility in Agricultural Systems Technology program. He is the PI in the MU Precision and Automated Agriculture Lab (https://cafnrfaculty.missouri.edu/mupaa). Dr. Zhou received PhD degree in Agricultural and Biological Engineering and worked as a Postdoctoral Research Associate at the Washington State University. He joined the University of Missouri as an Assistant Professor with a research-teaching appointment in 2016. Dr. Zhou’s research interest is mainly on precision agriculture, high-throughput crop phenotyping and robotic harvesting technologies using emerging sensors, UAV, internet of things, machine vision, robotics and artificial intelligence. At MU, his research activities focus on the development of emerging technologies for agricultural applications, including (i) Artificial intelligence (AI)-enabled decision-making systems based on unmanned aerial vehicle (UAV) remote sensing and advanced machine learning, (ii) Smart and digital agriculture technologies for crop and animal production, (iii) Robotic technologies for agricultural production, and (iv) Innovative technologies to improve safety and health of women farmers. Related research findings have been published in high-impact international journal papers and interested by industries. Dr. Zhou also authored five book chapters, edited two books and served as Associate Editor for Frontiers in Plant Science.
2. Canella Vieira, C., JN. Zhou, C. Cross, J. Heiser, B. Diers, D. E. Riechers, J. Zhou, D. H. Jarquin, H. T. Nguyen, G. Shannon, and P. Chen*. 2022. Differential responses of soybean genotypes to off-target dicamba damage. Crop Science. Online. https://doi.org/10.1002/csc2.20757
3. Vong, C. N., L. S. Conway, J. Zhou*, N. R. Kitchen and K. A. Sudduth. 2022. Corn stand uniformity estimation and mapping using UAV imagery and deep learning. Computers and Electronics in Agriculture. 198, 107008. https://doi.org/10.1016/j.compag.2022.107008
4. Canella Vieira, C., JN. Zhou, M. Usovsky, T. D. Vuong, H. Amanda, D. Lee, Z. Li, J. Zhou, J. G. Shannon, H. T. Nguyen, and P. Chen*. 2022. Exploring machine learning algorithms to unveil genomic regions associated with resistance to southern root-knot nematode in soybeans. Frontiers in Plant Science. 13, 883280. https://doi.org/10.3389/fpls.2022.883280
5. Canella Vieira, C., S. Sakar, F. Tian, JN. Zhou, D. Jarquin, H. T. Nguyen, J. Zhou, and P. Chen*. 2022. Differentiate soybean response to off-target dicamba damage based on UAV imagery and machine learning. Remote Sensing. 14(7), 1618. https://doi.org/10.3390/rs14071618 6. Feng, A., J. Zhou*, E. Vories, and K. Sudduth. 2022. Quantifying the effects of soil texture and weather on cotton development and yield using UAV imagery. Precision Agriculture. Online. https://doi.org/10.1007/s11119-022-09883-6
7. Zhou, JN., J. Zhou*, A. Scaboo, D. Yungbluth, and P. Chen. 2021. Soybean variety selection using UAV high-throughput phenotyping and machine learning. Frontiers in Plant Science. 12, 2543. https://doi.org/10.3389/fpls.2021.768742
8. Bernhardt, H., L. Schumacher, J. Zhou, M. Treiber, and K. Shannon. (2021). Digital Agriculture Infrastructure in the USA and Germany. Engineering Proceedings, 9(1), 1. https://doi.org/10.3390/engproc2021009001
9. Oseland, E., K. Shannon, J. Zhou, F. Fritschi, M. D. Bish, and K. W. Bradley. Evaluating the spectral response and yield of soybean following exposure to sublethal rates of 2,4-D and Dicamba at vegetative and reproductive growth stages. Remote Sensing. 2021(13), 3682. https://doi.org/10.3390/rs13183682
10. Zhou, JN., H. Mou, J. Zhou*, H. Ye, M.L. Ali, H. Nguyen, and P. Chen. 2021. Qualification of soybean responses to flooding stress using UAV-based imagery and deep learning. Plant Phenomics. 2021, 9892570. https://doi.org/10.34133/2021/9892570
11. Vong, C. N., L. S. Conway, J. Zhou*, N. R. Kitchen and K. A. Sudduth. 2021. Early corn stand count of different cropping systems using UAV-imagery and deep learning. Computers and Electronics in Agriculture. 186, 106214. https://doi.org/10.1016/j.compag.2021.106214
12. Vong, C. N., S. A. Stewart, J. Zhou*, N. R. Kitchen and K. A. Sudduth. 2021. Estimation of Corn Emergence Date Using UAV Imagery. Transactions of the ASABE, 64(4), 1173-1183. https://doi.org/10.13031/trans.14145
13. Fu, D., A. M. Scaboo, X. Niu, Q. Wang, and J. Zhou*. 2021. Non-destructive phenotyping fatty acid trait of single soybean seeds using reflective hyperspectral imagery. Journal of Food Process Engineering. 44(8), e13759. https://doi.org/10.1111/jfpe.13759
14. Zhou, S., H. Mou, JN. Zhou, J. Zhou*, H. Ye and H. Nguyen. 2021. Development of an automated plant phenotyping system for evaluation of salt tolerance in soybean. Computers and Electronics in Agriculture. 182, 106001. https://doi.org/10.1016/j.compag.2021.106001
15. Zhou, JN., J. Zhou*, H. Ye, M. L. Ali, H. Nguyen, and P. Chen. 2021. Yield estimation of soybean breeding lines using UAV multispectral imagery and convolutional neuron network. Biosystems Engineering. 204, 90-103. https://doi.org/10.1016/j.biosystemseng.2021.01.017
16. Feng, A., J. Zhou*, E. Vories, and K. Sudduth. 2020. Evaluation of cotton emergence using UAV-based imagery and deep learning. Computers and Electronics in Agriculture. 177, 105711. https://doi.org/10.1016/j.compag.2020.105711
17. Feng, A., J. Zhou*, E. Vories, and K. Sudduth. 2020. Evaluation of cotton emergence using UAV-based narrow-band spectral imagery with customized image alignment and stitching algorithms. Remote Sensing, 12(11), 1764. https://doi.org/10.3390/rs12111764
18. Zhou, JN., J. Zhou*, H. Ye, M. L. Ali, H. Nguyen, and P. Chen. 2020. Classification of soybean leaf wilting due to drought stress using UAV-based imagery. Computers and Electronics in Agriculture. 175, 105576. https://doi.org/10.1016/j.compag.2020.105576
19. Feng, A., M. Zhang, K. Sudduth, E. Vories, and J. Zhou*. 2020. Yield estimation in cotton using UAV-based multi-sensor imagery. Biosystems Engineering. 193, 101-114. https://doi.org/10.1016/j.biosystemseng.2020.02.014
20. Zhang, M., J. Zhou*, K. A. Sudduth, and N. R. Kitchen. 2020. Estimation of maize yield and effects of variable-rate nitrogen application using UAV-based RGB imagery. Biosystems Engineering. 189, 24-35. https://doi.org/10.1016/j.biosystemseng.2019.11.001
21. Zhou, JN., D. Yungbluth, C. N. Vong, A. Scaboo, and J. Zhou*. 2019. Estimation of the maturity date of soybean breeding lines using UAV-based multispectral imagery. Remote Sensing. 11(8), 2075. https://doi.org/10.3390/rs11182075
22. Cao, W., JN. Zhou, Y. Yuan, H. Ye, H. Nguyen, J. Chen, and J. Zhou*. 2019. Quantifying variation in soybean due to flood using a low-cost 3D imaging system. Sensors. 19(12), 2682. https://doi.org/10.3390/s19122682
23. Zhang, M.*, A. Feng, J. Zhou, and X. Lv. 2019. Cotton yield prediction using remote visual and spectral images captured by UAV system. Transactions of the Chinese Society of Agricultural Engineering. 35(5), 91-98. https://doi.org/10.11975/j.issn.1002-819.2019.05.011
24. Zhou, JN., X. Fu, S. Zhou, J. Zhou*, H. Ye, H. Chen, and H. Nguyen. 2019. Automated segmentation of soybean plants in greenhouse from 3D imagery point cloud using machine learning methods. Computers and Electronics in Agriculture. 162, 143-153. https://doi.org/10.1016/j.compag.2019.04.014
25. Feng, A., M. Zhang, K. Sudduth, E. Vories, and J. Zhou*. 2019. Cotton yield estimation from UAV-based plant height. Transactions of the ASABE. 62(2). https://doi.org/10.13031/trans.13067
26. Zhou, JN., X. Fu, L. Schumacher, and J. Zhou*. 2018. Evaluating geometric measurement accuracy based on 3D reconstruction of automated imagery in greenhouse. Sensors. 18(7), 2270-2286. https://doi.org/10.3390/s18072270
27. Zhou, J.*, H. Chen, JN. Zhou, X. Fu, H. Ye, H. Nguyen. 2018. Develop an automated phenotyping platform for quantifying soybean dynamic responses to salinity stress in greenhouse environments. Computers and Electronics in Agriculture. 151, 319-330. https://doi.org/10.1016/j.compag.2018.06.016
28. Ranjan, R., A. Chandel, L. Khot*, H. Bahlol, J. Zhou, R. Boydston, and P. Miklas. 2019. Irrigated pinto bean crop stress and yield assessment using ground based low altitude remote sensing technology. Information Processing in Agriculture. 6(4): 502-514. https://doi.org/10.1016/j.inpa.2019.01.005
29. Zhang, C., M. Pumphrey, J. Zhou, Q. Zhang, and S. Sankaran*. 2018. Development of automated high-throughput phenotyping system for wheat evaluation in controlled environment. Transactions of the ASABE. 62(1):61-74. https://doi.org/10.13031/trans.12856.
30. Sankaran, S.*, J. Zhou, P. Miklas. 2018. High-throughput field phenotyping in dry bean using small unmanned aerial vehicle based multispectral imagery. Computers and Electronics in Agriculture. 151, 84-92. https://doi.org/10.1016/j.compag.2018.05.034.
31. Zhou, J., L. Khot, R. A*. Boydston, P. N. Miklas and L. Porter. 2018. Low altitude remote sensing technologies for crop stress monitoring: a case study on spatial and temporal monitoring of irrigated pinto bean. Precision Agriculture. 19(3), 555-569. https://doi.org/10.1007/s11119-017-9539-0.
32. Zhou, J., L. Khot*, H. Bahlol, G. Kafle, T. Peters, M. D. Whiting, Q. Zhang, and D. Granatstein. 2017. In-field sensing for crop loss management: efficacy of air-blast sprayer generated crosswind in rainwater removal from cherry canopies. Crop Protection. 91 (2017), 27-33. https://doi.org/10.1016/j.cropro.2016.09.010.
33. Zhou, J., L. He, Q. Zhang*, and M. Karkee. 2016. Field Evaluation of a mechanical-assist cherry harvesting system. Engineering in Agriculture, Environment and Food. 9(4), 324-331. https://doi.org/10.1016/j.eaef.2016.05.003.
34. Zhou, J., M. J. Pavek, S. C. Shelton, Z. J. Holden, and S. Sankaran*. 2016. Aerial multispectral imaging for crop hail damage assessment in potato. Computers and Electronics in Agriculture. 127(2016), 406-412. https://doi.org/10.1016/j.compag.2016.06.019.
35. Zhou, J., L. Khot*, T. Peters, M. D. Whiting, Q. Zhang, and D. Granatstein. 2016. Efficacy of unmanned helicopter in rainwater removal from cherry canopies. Computers and Electronics in Agriculture. 124(2016), 161-167. https://doi.org/10.1016/j.compag.2016.04.006.
36. Kafle, G., L. Khot*, J. Zhou, H. Bahlol, and Y. Si. 2016. Towards precision spray applications to prevent rain-induced sweet cherry cracking: understanding calcium washout due to rain and fruit cracking susceptibility. Scientia Horticulturae. 203(2016), 152-157. https://doi.org/10.1016/j.scienta.2016.03.027.
37. Trapp, J. J., C. A. Urrea, J. Zhou, L. R. Khot, S. Sankaran, and P. N. Miklas*. 2016. Selective phenotyping traits related to multiple stress and drought response in dry bean. Crop Science. 56(2016), 1-13. https://doi.org/10.2135/cropsci2015.05.0281.
38. Wang, M., P. Ellsworth, J. Zhou, A. Cousins, S. Sankaran*. 2016. Evaluation of water-use efficiency in foxtail millet (Setaria italica) using visible-near infrared and thermal spectral sensing techniques. Talanta. 152(2016), 531-539. https://doi.org/10.1016/j.talanta.2016.01.062.
39. Zhou, J., L. He, Q. Zhang*, and M. Karkee. 2016. Analysis of shaking-induced cherry fruit motion and damage. Biosystems Engineering. 144(2016): 105-114. https://doi.org/10.1016/j.biosystemseng.2016.02.007.
40. Zhou, J., L. He, Q. Zhang*, and M. Karkee. 2016. Effect of catching surface and tilt angle on bruise damage of sweet cherry due to mechanical impact. Computers and Electronics in Agriculture. 121(2016), 282-289. https://doi.org/10.1016/j.compag.2016.01.004. 41. He, L., J. Zhou, Q. Zhang*, and H. J. Charvet. 2016. A string twining robot for high-trellis hop production. Computers and Electronics in Agriculture. 121(2016), 207-214. https://doi.org/10.1016/j.compag.2015.12.012. 42. He, L., J. Zhou, Q. Zhang*, and M. Karkee. 2015. Evaluation of multi-pass mechanical harvesting on ‘Skeena’ sweet cherries. HortScience. 50(8), 1178-1182. https://doi.org/10.21273/HORTSCI.50.8.1178.
43. Zhou, J., L. He, Q. Zhang*, and M. Karkee. 2014. Effect of excitation position of a handheld shaker on fruit removal efficiency and damage in mechanical harvesting of sweet cherry. Biosystems Engineering. 125(2014): 36-44. https://doi.org/10.1016/j.biosystemseng.2014.06.016.
44. Zhou, J., L. He, Q. Zhang*, X. Du, D. Chen, and M. Karkee. 2013. Evaluation of the influence of shaking frequency and duration in mechanical harvest of sweet cherry. Applied Engineering in Agriculture. 29(5): 607-612. https://doi.org/10.13031/aea.29.9917.
45. He, L., J. Zhou, X. Du, D. Chen, Q. Zhang*, and M. Karkee. 2013. Energy efficacy analysis of a mechanical shaker in sweet cherry harvesting. Biosystems Engineering. 116(4): 309-315. https://doi.org/10.1016/j.biosystemseng.2013.08.013.
46. Khot, A. L., and J. Zhou. 2016. Precision agriculture: beyond the domain of small UAS. ASABE Resource Magazine, 23(3), 201-21.
BOOK CHAPTER
1. Zhou, JN., C. N. Vong, J. Zhou*. 2021. Imaging Technology for High-Throughput Plant Phenotyping. In Sensing, data managing, and control technologies for agricultural systems. Springer, Cham.
2. Feng, A., C. N. Vong, J. Zhou*. 2021. Unmanned aerial vehicle (UAV) applications in cotton production. In Progress and advances of unmanned aerial systems (UASs) in agricultural applications. Springer, Cham.
3. Zhou, J.*, H. Nguyen. 2021. Solve the breeder’s equation using high-throughput crop phenotyping technology. In: Zhou J., Nguyen H.T. (eds) High-throughput Crop Phenotyping, Ch1. Springer, Cham. https://doi.org/10.1007/978-3-030-73734-4_1.
4. Zhou, J.*, JN. Zhou, H. Nguyen, H. Ye. 2021. High-throughput crop phenotyping systems for controlled environments. In: Zhou J., Nguyen H.T. (eds) High-Throughput Crop Phenotyping. Concepts and Strategies in Plant Sciences. Springer, Cham. https://doi.org/10.1007/978-3-030-73734-4_9
5. Chen, H., H. Ye, T. Do, J. Zhou, B. Valliyodan, G. Shannon, P. Chen, X. Chen, and H. Nguyen. 2019. Advances in Genetics and Breeding of Salt Tolerance in Soybean. In: Kumar V., Wani S., Suprasanna P., Tran LS. (eds) Salinity Responses and Tolerance in Plants, Volume 2. Springer, Cham. https://doi.org/10.1007/978-3-319-90318-7_9
BOOK EDITOR
1. Zhou, J, Nguyen, H. (editors). 2021. Advanced Concepts and strategies in Plant Sciences: High-throughput Crop Phenotyping. Springer. https://doi.org/10.1007/978-3-030-73734-4.
2. Zhang, Z., H. Liu, C. Yang, Y. Ampatzidis, J. Zhou and Y. Jiang (editors). 2022. Unmanned Aerial Systems in Precision Agriculture: Technological Progresses and Applications. Springer, Singapore. https://doi.org/10.1007/978-981-19-2027-1
